Customer feedback is a core piece of customer experience management (CXM). The high volume of data is one of the main challenges of feedback management. It limits the benefits businesses could achieve from customer feedback insights. It is almost impossible to examine every piece of customer feedback without the right tools. Let alone generate relevant insights that can drive business impact.
But fear not. Text analytics is here to the rescue. Text analytics analyzes large volumes of unstructured text data to uncover meaningful insights. It can process customer feedback from different sources, including online reviews, social media comments, and survey responses. Text analytics helps you understand what’s beneath customer feedback, positive or negative. Better yet, it reveals customer sentiment, patterns, and trends for more data-driven decisions.
For businesses getting started with text analytics, it’s important to understand the factors that can ensure its successful implementation. In this article, we explore the core elements of text analytics and share eight best practices for CX leaders.
Importance of Collecting and Analyzing Open-Ended Feedback
Open-ended feedback refers to responses from customers that are not limited by predefined options or multiple-choice answers. Instead, customers are encouraged to express their thoughts, opinions, and experiences in their own words. This type of feedback is often collected through surveys, reviews, social media comments, and customer support interactions.
Benefits of Open-Ended Feedback
Open-ended feedback provides a rich source of information that goes beyond numerical ratings or yes/no answers. It allows customers to elaborate on their experiences, offering nuanced insights into their needs, preferences, and pain points. This qualitative data can reveal underlying emotions, motivations, and detailed suggestions for improvement, providing a deeper understanding of the customer journey.
Challenges of Analyzing Open-Ended Feedback
While open-ended feedback is valuable, analyzing it manually can be a daunting task. The sheer volume of responses can be overwhelming, making it difficult to identify patterns or trends. Without text analytics, businesses may struggle to systematically process and interpret this data, leading to missed opportunities and insights. Manual analysis is also prone to bias and inconsistency, further complicating the extraction of actionable insights.
Using Text Analytics to Process and Interpret Open-Ended Feedback
Text analytics plays a crucial role in overcoming the challenges of analyzing open-ended feedback. By leveraging techniques such as Natural Language Processing (NLP), sentiment analysis, and text mining, businesses can efficiently process large volumes of text data. Text analytics tools can identify key themes, sentiments, and trends, enabling businesses to understand customer feedback at scale. This technology transforms qualitative data into quantifiable insights, facilitating data-driven decision-making and continuous improvement in customer experience.
The Basic Elements of Text Analytics
Text analytics involves the use of advanced technologies to analyze unstructured text data. It combines various techniques and methodologies designed to extract meaningful information from textual content. The primary components of text analytics include Natural Language Processing (NLP), sentiment analysis, and text mining.
Natural Language Processing (NLP)
Natural Language Processing (NLP) is a branch of artificial intelligence that enables computers to understand, interpret, and respond to human language. NLP techniques are used to process and analyze large amounts of natural language data. This involves tasks such as tokenization, part-of-speech tagging, named entity recognition, and syntactic parsing. NLP helps in extracting structured information from unstructured text, making it easier to analyze and derive insights.
Sentiment Analysis
Sentiment analysis, also known as opinion mining, is a technique used to determine the emotional tone behind a piece of text. It identifies whether the sentiment expressed is positive, negative, or neutral. Sentiment analysis helps businesses gauge customer emotions and reactions, providing insights into customer satisfaction and overall sentiment toward products or services. This information is valuable for identifying areas of improvement and addressing customer concerns promptly.
Text Mining
Text mining involves extracting valuable information from large volumes of text data. It encompasses techniques such as keyword extraction, topic modeling, and document clustering. Text mining helps in uncovering hidden patterns, trends, and relationships within the text. Using text mining in customer feedback helps identify common themes and recurring issues, enabling businesses to prioritize actions and make informed decisions.
The Role of Context in Text Analytics
Context is critical in text analytics as it ensures the accuracy and relevance of the insights derived. Understanding the context in which feedback is given helps in accurately interpreting the meaning and sentiment behind the words. Context-specific text analytics models are tailored to the unique language and nuances of different industries or business domains, providing more precise and actionable insights compared to generic models. This contextual understanding is essential for delivering a personalized and effective customer experience.
8 Text Analytics Best Practices in CXM
1. Have Clear Objectives
The first step to implementing text analytics is to define specific goals. Determine what you want to achieve, whether it’s improving customer satisfaction, identifying pain points, or enhancing product features. Clear objectives help in selecting the right tools and techniques, ensuring that your text analytics efforts align with your overall business goals and provide actionable insights.
2. Collect Comprehensive Customer Feedback
Gathering a wide range of feedback is essential for effective text analytics. Use multiple channels such as surveys, social media, reviews, and customer support interactions to collect diverse and comprehensive customer input. Real-time feedback collection methods can provide timely insights and help businesses respond quickly to customer needs. The more varied and rich your data sources, the better your analysis will be.
3. Choose the Right Text Analytics Tools
Selecting the appropriate text analytics tools is critical for success. Look for tools that offer robust Natural Language Processing (NLP) capabilities, sentiment analysis, and text mining features. Evaluate the tools based on their ease of use, integration capabilities with your existing systems, and scalability. Choosing the right tools ensures efficient processing and accurate analysis of customer feedback.
4. Leverage Sentiment Analysis
Sentiment analysis is a powerful technique for understanding customer emotions and reactions. Implement sentiment analysis to gauge the overall sentiment of customer feedback, identify areas of concern, and track changes in sentiment over time. Accurate sentiment analysis helps businesses understand customer satisfaction levels and address negative feedback promptly. By leveraging sentiment analysis, companies can improve customer relationships and enhance their CX strategies.
5. Utilize Advanced NLP Techniques
Advanced NLP techniques enable deeper understanding and more precise interpretation of customer feedback. Techniques such as topic modeling, and syntactic parsing help in extracting valuable insights from unstructured text. Implementing these NLP techniques can uncover hidden patterns, detect emerging trends, and provide a more nuanced understanding of customer needs ensuring that businesses get the most out of their text analytics efforts.
6. Integrate Text Analytics with Other CX Data
For a holistic view of customer experience, integrate text analytics with other CX data such as quantitative survey results, transactional data, and behavioral data. Combining different data sources provides a comprehensive understanding of the customer journey and enables more accurate identification of pain points and opportunities. Integrated data analysis helps in developing well-rounded strategies for improving customer experience.
7. Implement Continuous Improvement Processes
Text analytics should not be a one-time effort. Establish continuous improvement processes to regularly analyze and act on customer feedback. Monitor the effectiveness of your text analytics initiatives and refine your strategies based on the insights gained. Regularly updating and improving your text analytics models ensures that they remain relevant and effective in addressing evolving customer needs. Continuous improvement fosters a culture of responsiveness and agility in CXM.
8. Ensure Data Privacy and Compliance
Protecting customer data and ensuring compliance with relevant regulations should be among the top priorities of every customer-centric business. Implement robust data privacy measures and follow best practices for data security. Be transparent with customers about how their data is used and ensure compliance with regulations such as GDPR and CCPA. This care with privacy and compliance will build trust with customers and safeguard brand reputation.
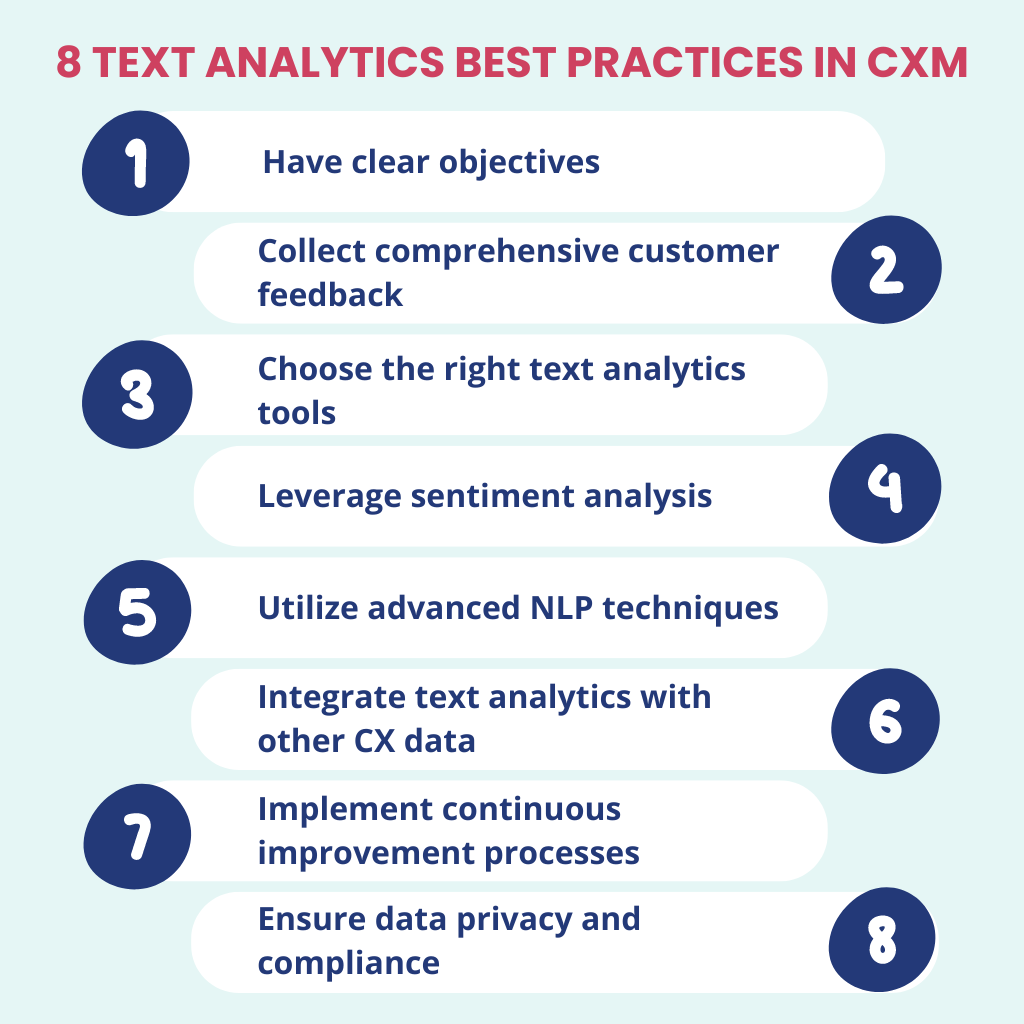
By continuously analyzing and acting on customer feedback, companies can drive meaningful improvements in their customer experience initiatives. Implementing text analytics in customer experience management is a powerful strategy to gain deeper insights into customer feedback and enhance overall satisfaction. By following these best practices businesses can achieve the full potential of text analytics. Businesses that invest in these technologies and prioritize customer-centric strategies will be well-positioned to thrive in an increasingly customer-driven world.
Did you like the post?
You might also like:
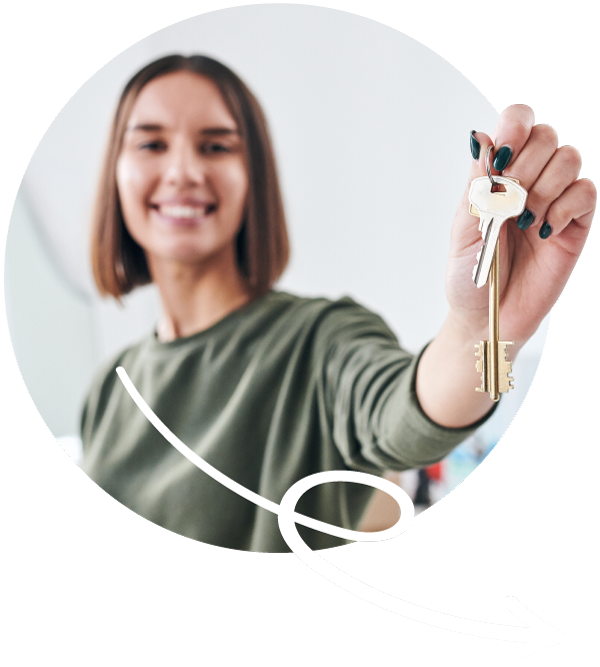
Surveypal
Everything you need to lead and improve your customer experience. Learn more at surveypal.com, or